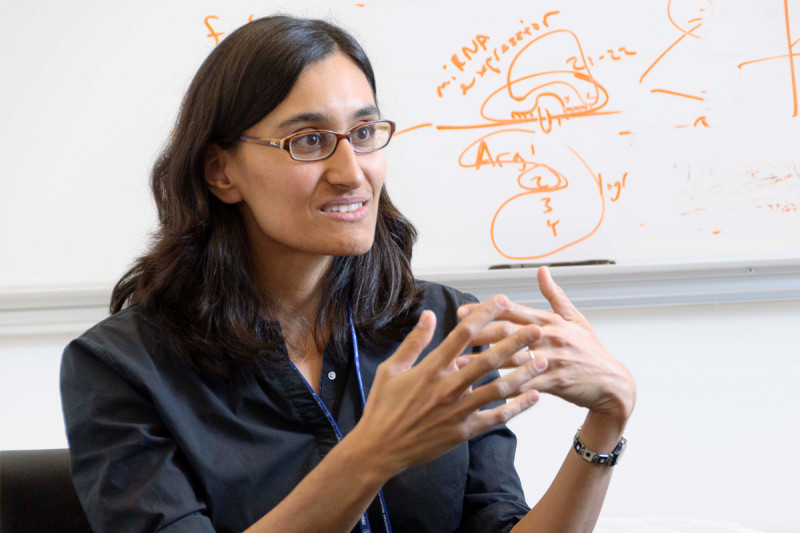
Christina Leslie
Computational biologist Christina Leslie uses computational methods to exploit vast amounts of biological data. Her research is focused on learning how genes are expressed in regular cells and what goes wrong when gene expression gets dysregulated in cancer cells. In 2013, Dr. Leslie discussed with us how computational science can help solve the complex problems of cancer biology.
Computational biology is a broad field, but a short explanation for what most computational biologists do is that we use computational methods to help make sense of very large amounts of biological data. I trained as a mathematician, and mathematicians are often attached to finding elegant solutions to problems.
In biology, our focus instead is on deriving meaningful insights into biological processes. So while we still like to design clever algorithms to solve problems, the most important thing is asking the right question in the first place.
Leaps in Technology
For most of the history of modern biology, scientists have studied one gene at a time. In the late 1990s, it became possible to simultaneously measure the expression of thousands of genes using microarrays (also known as DNA chips), giving a kind of genome-wide “snapshot” of gene activity in each sample of cells.
More recently, there has been another technological revolution, called next-generation sequencing, and now biologists are rapidly amassing even more comprehensive data. For example, for each tumor sample that we study, RNA sequencing produces millions of short sequences (“reads”) that we match to the genome and analyze statistically. (Like DNA, RNA is a large biological molecule consisting of strings of nucleotides; RNAs are transcribed from the genome using regions of DNA as a template.) This statistical analysis allows us to measure changes in expression for thousands of protein-coding genes as well as RNAs that don’t code for proteins, to detect alterations in the way these genes were processed and to map their mutations.
Learning from Data to Make Predictions
My lab develops computational and statistical methods to exploit this data in order to study how genes are expressed in regular cells and to learn what goes wrong when gene expression gets dysregulated in cancer cells.
The methods we use often come from machine learning. These are algorithms that “learn” from data to build a model that can be used to make accurate predictions. Many people have interacted with machine learning applications, perhaps without realizing it. For example, when you use a digital camera to take a picture, face detection software locates the faces in the field of view to allow the camera to focus better. The face detector was “trained” on a big data set of photos where the locations of faces were known, so that it could learn to discriminate between face and non-face patches of photos. It can then be applied to new data — in this case, new faces in the photo you want to take — and accurately predict the faces.
In our work, rather than faces, the data sets are made up of large quantities of data derived from different kinds of sequencing or microarray technologies. Often our models are designed to predict how genes are regulated. In one project, we are trying to train a model that will teach us more about the mechanism of gene silencing — a process that is dysregulated in many diseases, including cancer — using small pieces of RNA called microRNAs. In another project, we are trying to decode the information in regulatory regions of the genome that govern differentiation of stem cells into fully specialized cell types.
As a computational scientist, I came to Memorial Sloan Kettering because I wanted to be immersed in an exciting biomedical science environment and work on important problems in cancer biology. This is an amazing place because scientists are encouraged to be ambitious and tackle big questions — which sometimes involves generating huge and complex data sets. Through close collaboration with experimental labs here, we have started to leverage the power of computational and machine learning methods to advance cancer research