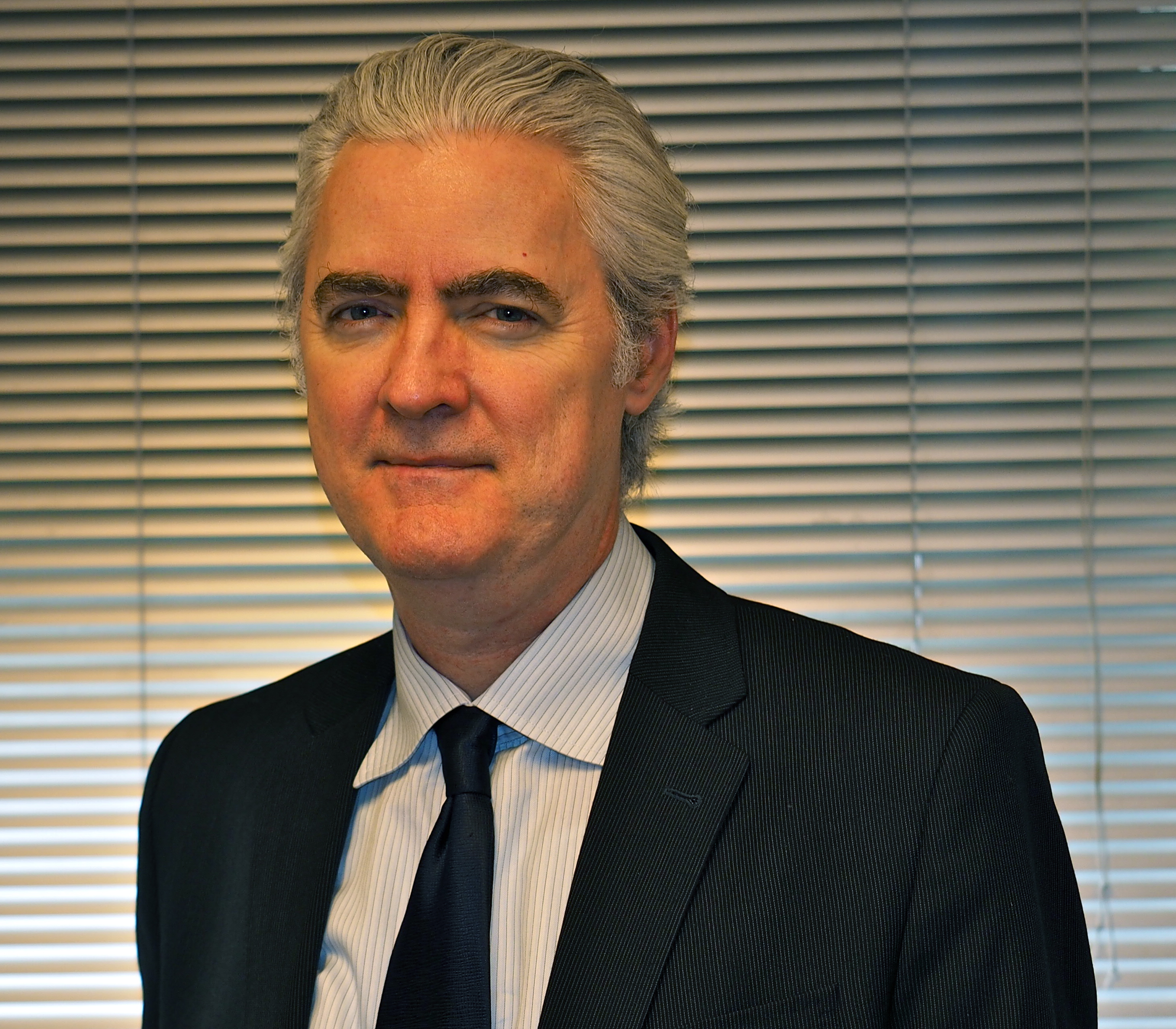
Chair of Medical Physics, Chief of the Service for Predictive Informatics, Attending Physicist
Dr. Deasy is a widely published author in medical physics who has written on image segmentation, proton beam treatment planning and optimization, intensity-modulated radiotherapy treatment planning, predictive modeling of tumor and normal tissue response based on multi-institutional datasets, Monte Carlo dose calculation methods, open-source research software development, and the identification of biomarkers to predict treatment response.
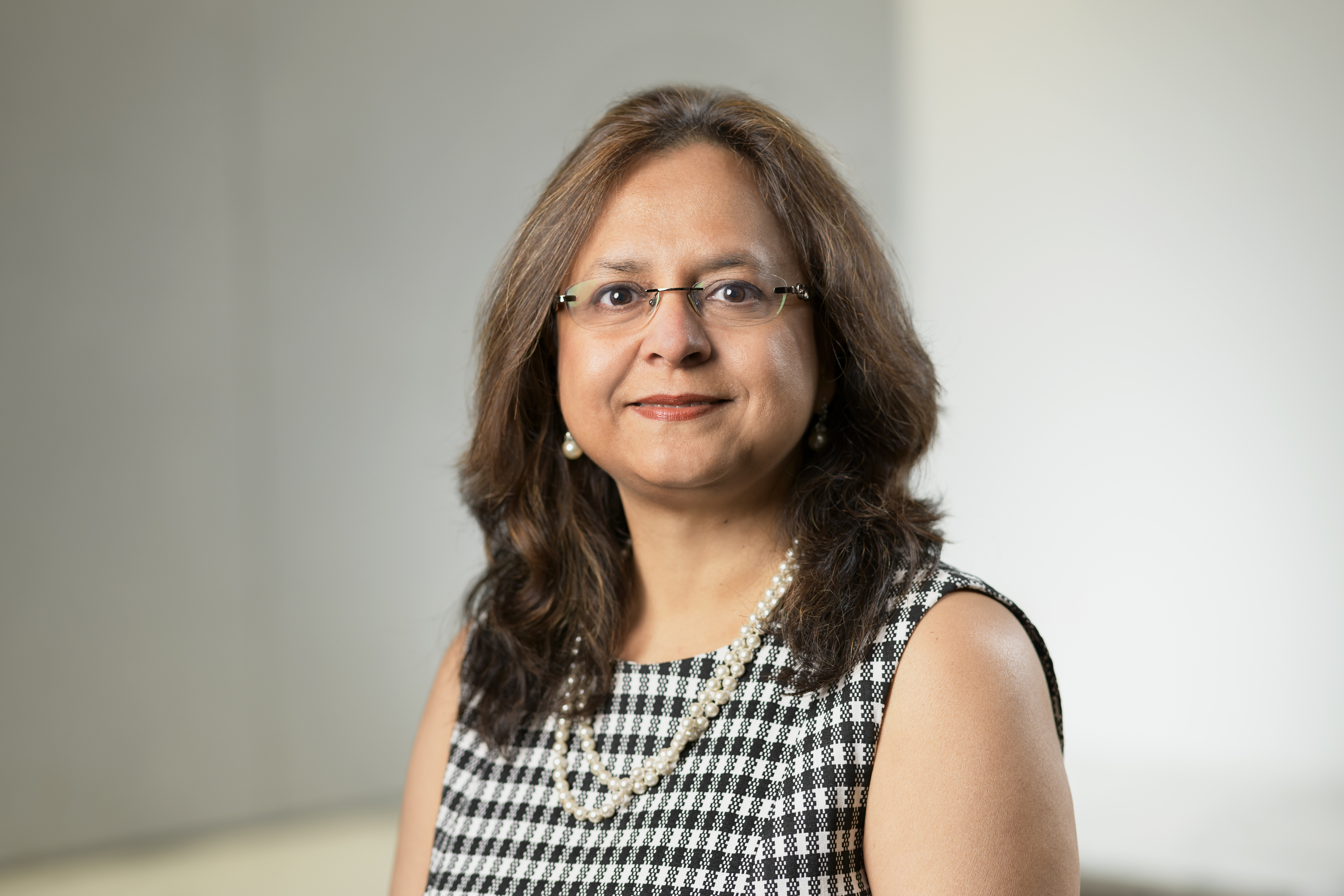
Deputy Service Chief, Predictive Informatics, Attending Physicist
Dr. Shukla-Dave is the Deputy Chief for the Service of Predictive Informatics and Director of Quantitative Imaging. In these capacities, her overarching goal is to build a strong, world-reputed “Quantitative Imaging” program at MSK that encompasses faculty across Medical Physics, Radiology, Radiation Oncology, and other departments, with a strong interest in enriching the clinical imaging at the Center. This involves creating an infrastructure that provides tools and expertise to standardize imaging protocols and metrics, implements new and novel imaging methods to be in the forefront of oncologic imaging, and provides an environment to mentor younger faculty and fellows in Quantitative Imaging. Dr. Shukla-Dave is also harmonizing her combined efforts with those of external agencies in which she is an active member such as the National Cancer Institute (NCI)/Quantitative Imaging Network (QIN), Radiological Society of North America (RSNA)/Quantitative Imaging Biomarker Alliance (QIBA), and International Society of Magnetic Resonance in Medicine (ISMRM)/Standards in Quantitative MR (SQMR).
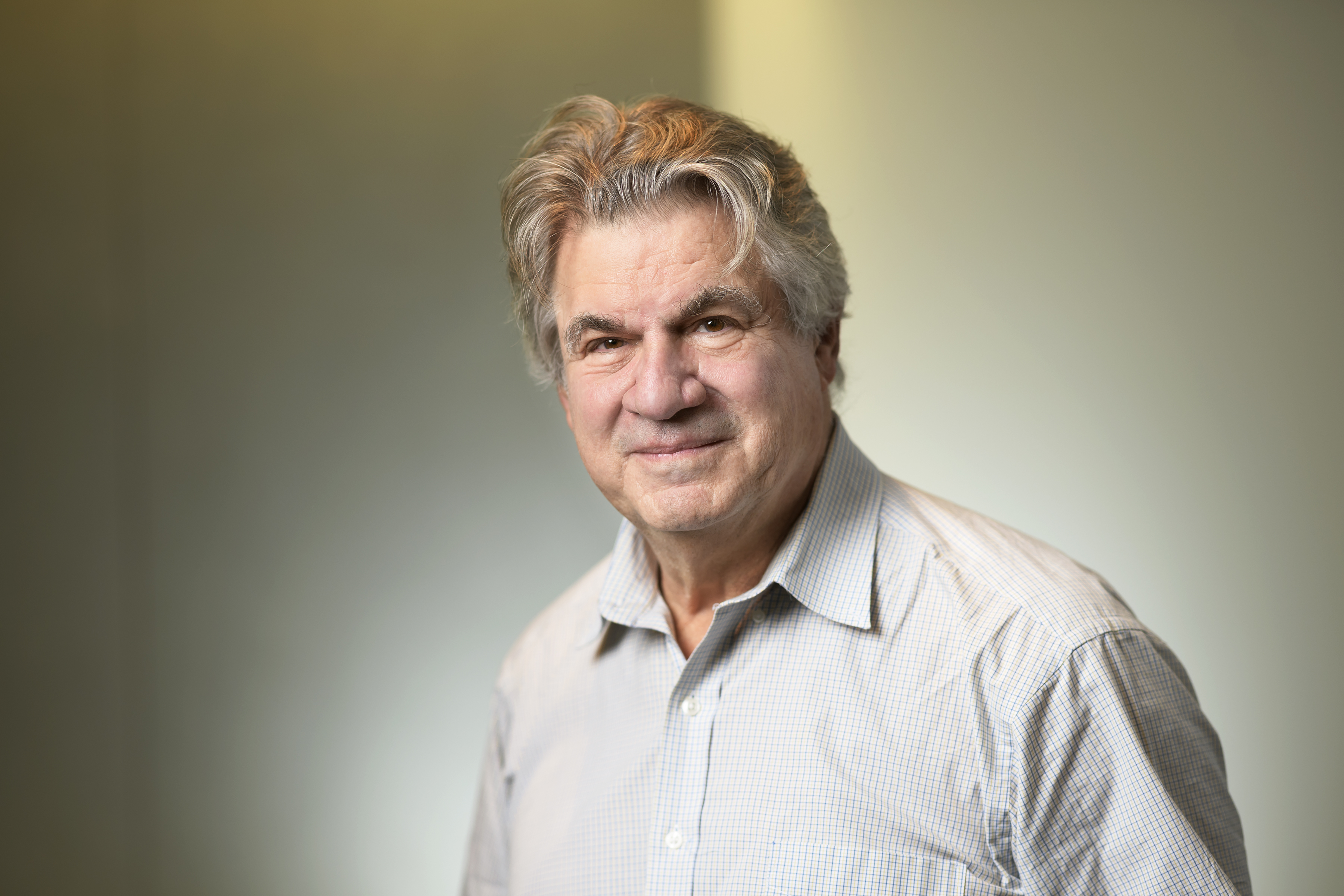
Distinguished Professor of Computer Science and Applied Mathematics & Statistics at Stony Brook University, Affiliate Attending in the Service of Predictive Informatics at MSK
Dr. Tannenbaum is an applied mathematician with extensive experience in electrical and biomedical engineering as well as computer science. He has almost four decades of experience working in systems, computer vision, image processing, and medical imaging computation. He has graduated 46 doctoral and 21 master’s students as well as supervised or co-supervised 18 postdoctoral fellows.
Dr. Tannenbaum has been involved in several NIH sponsored efforts regarding the latter subject, including as a site PI of the Algorithms Core of the U54 National Alliance of Medical Image Computing (NA-MIC) as well as the P41 Neuroanalysis Center both at the Harvard Medical School. Presently, he is supported on a NIA R01 for the study of the glymphatic system and neurodegenerative diseases. Dr. Tannenbaum performed pioneering work in the 1980s and 1990s on robust control. More recently, he has developed novel methodologies for segmentation, registration, and visualization, many of which are now included in the 3D SLICER software package. He has been applying methods from mathematical network analysis to various biological problems, including cancer networks. He has also performed extensive research in visual tracking and controlled active vision supported by the Department of Defense (DoD) and the National Science Foundation (NSF).
Dr. Tannenbaum formulated a new approach to optimal mass transport (Monge-Kantorovich) theory in joint work with Steven Haker and Sigurd Angenent. In 2001, Haker and Tannenbaum proposed a method of elastic registration based on the Monge-Kantorovich problem of optimal mass transport. Their method for finding the registration mapping is based on a partial differential equation approach to the minimization of the L 2 Kantorovich–Wasserstein or “Earth Mover’s Distance” under a mass preservation constraint. They showed how this approach leads to practical algorithms and demonstrated their method with several examples. They also extended this method to consider changes in intensity and showed that it is well suited for applications such as image morphing. Dr. Tannenbaum has recently developed techniques using graph curvature ideas for analyzing the robustness of complex networks. These ideas have wide applicability and will be used in integrative analysis.
Assistant Attending Physicist
Dr. Apte has a wide range of experience in radiotherapy informatics. He has been the lead developer of various open-source informatics systems to facilitate outcomes research. He collaborates with multiple radiotherapy research groups from academia and industry on informatics projects. He is currently involved in developing data pooling tools that will help to build and verify tumor control probability and normal tissue complications probability models based on dose-volume, clinical, demographic, and genomic variables.
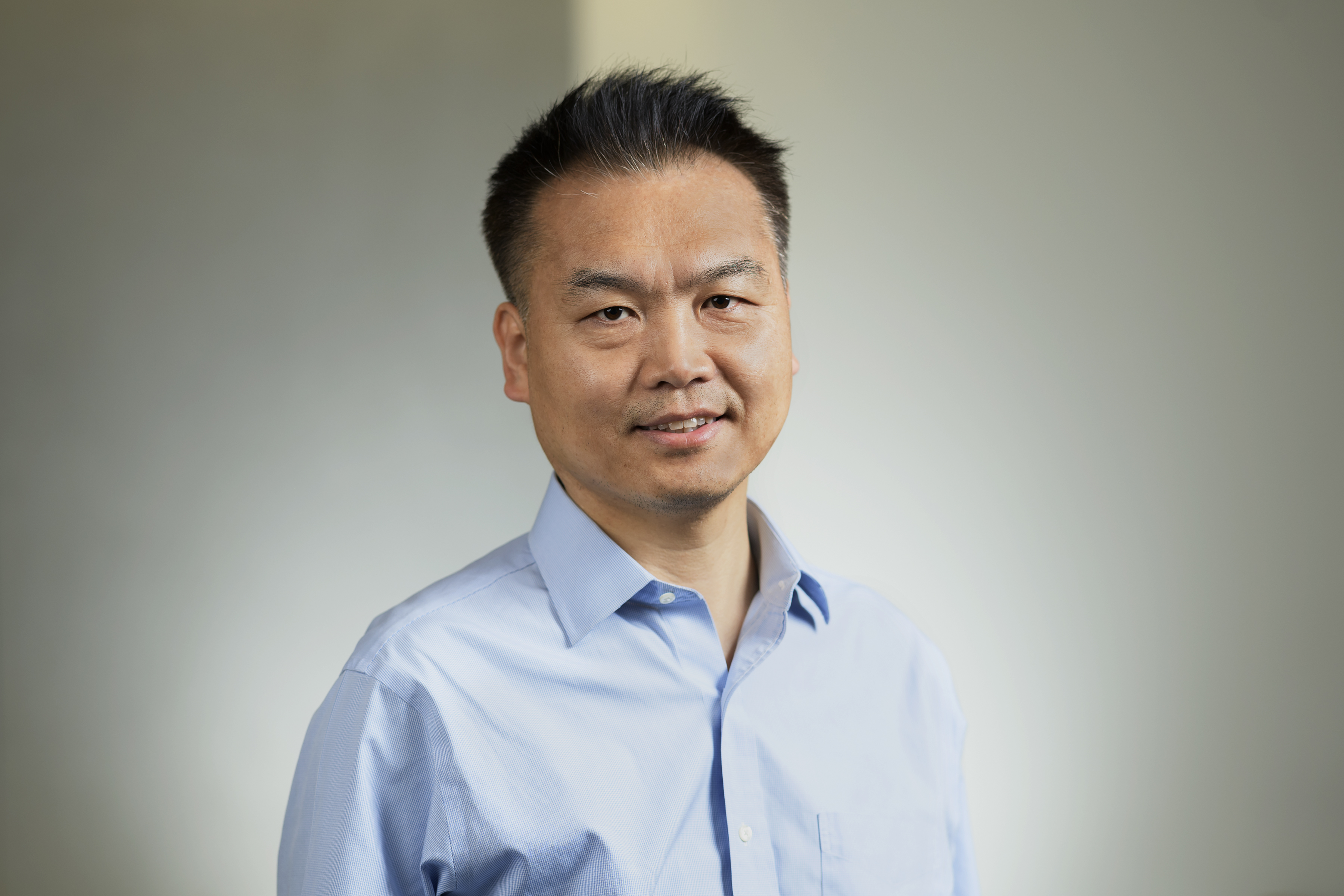
Assistant Attending Computer Scientist
Dr. Oh works on several methods of identifying novel biomarkers and building predictive models of outcome in radiation oncology using bioinformatics and machine learning techniques. His research strives to use cutting-edge computational and statistical methods, based on bioinformatics and machine learning techniques, to improve the treatment of cancer.
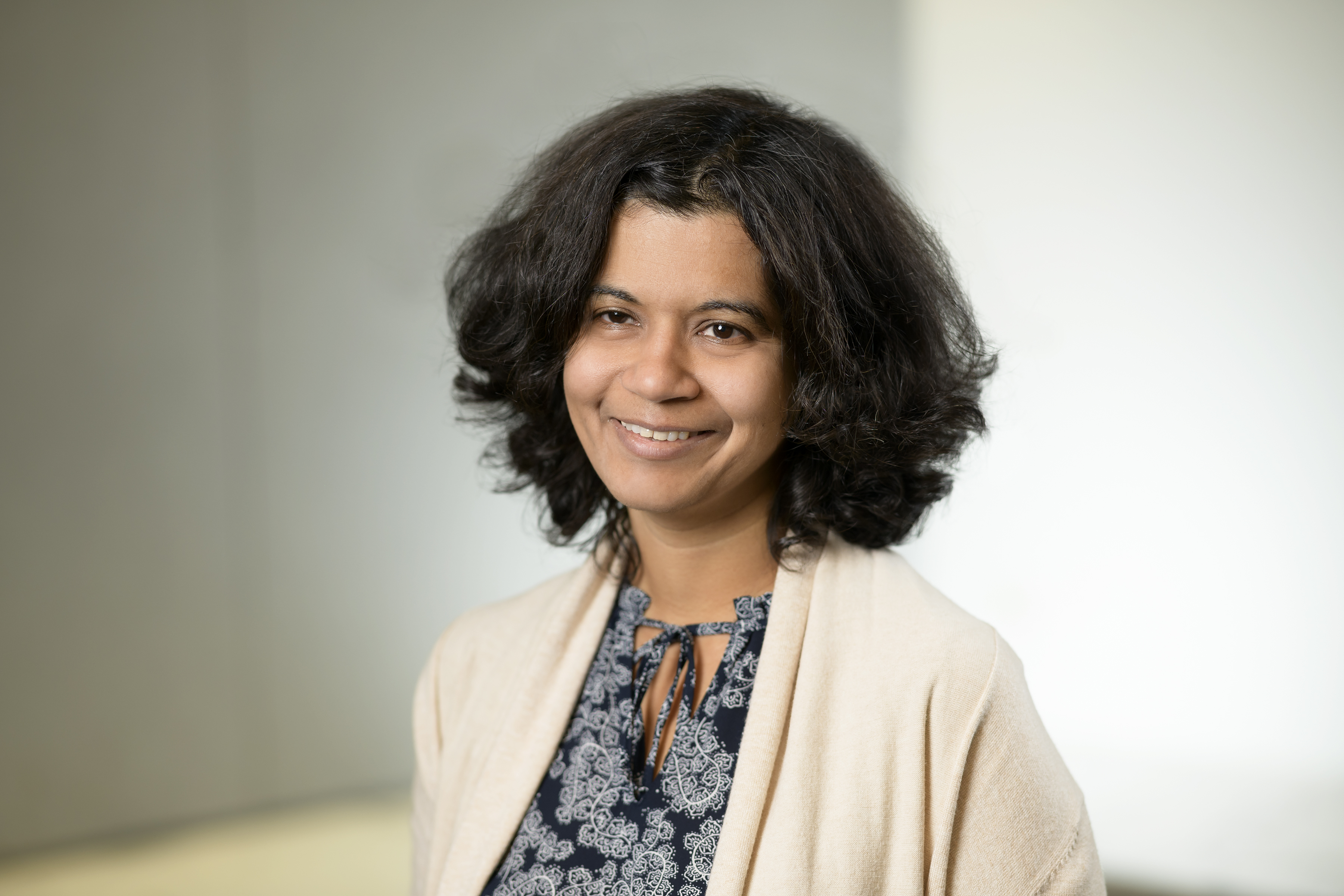
Assistant Attending Computer Engineer
Dr. Veeraraghavan develops computer algorithms and software for computer-aided medical image analysis. Her work focuses on combining techniques from machine learning and estimation theory with computer vision methods to solve difficult problems in automated and semi-automated image segmentation, combining multiple imaging modalities and sequences for segmentation, tissue–tumor classification, and image registration.
Chief of Magnetic Resonance Imaging and Spectroscopy Physics, Associate Member in Medical Physics and Radiology
Dr. Otazo is one of the early developers of compressed sensing techniques for MRI and continues to be a leader in the field. His group’s compressed sensing techniques, such as k-t SPARSE-SENSE, GRASP, XD-GRASP and L+S, have made seminal contributions and were successfully applied to a wide range of clinical imaging problems to improve speed and motion-robustness and provide comprehensive and quantitative information. More recently, he has applied similar compressed sensing ideas to reduce radiation dose in CT and developed the first prototype for data undersampling in clinical CT scanners. He has broad expertise in the development of image reconstruction algorithms for medical imaging. His most recent interest is the application of machine learning techniques to image reconstruction.
Lead Medical Physicist
Mr. Mahmood, an American College of Radiology board certified diagnostic medical physicist, has a broad background in medical physics, including training and expertise in data science, machine learning, and quantitative analysis of medical image quality. His current research activities focus on methods to validate quantitative imaging features that are used to objectively characterize the tumor microenvironment and to develop large-scale datasets for the purposes of training machines to accurately classify pancreatic cancer found on CT scans. Recently, with the assistance of a summer student who he successfully mentored, he laid the groundwork for the first aim of TR&D 3 by developing a 3D printed phantom that replicates the complex anatomical shape, micro-texture and radio-opaqueness of tumors detected on CT scans.
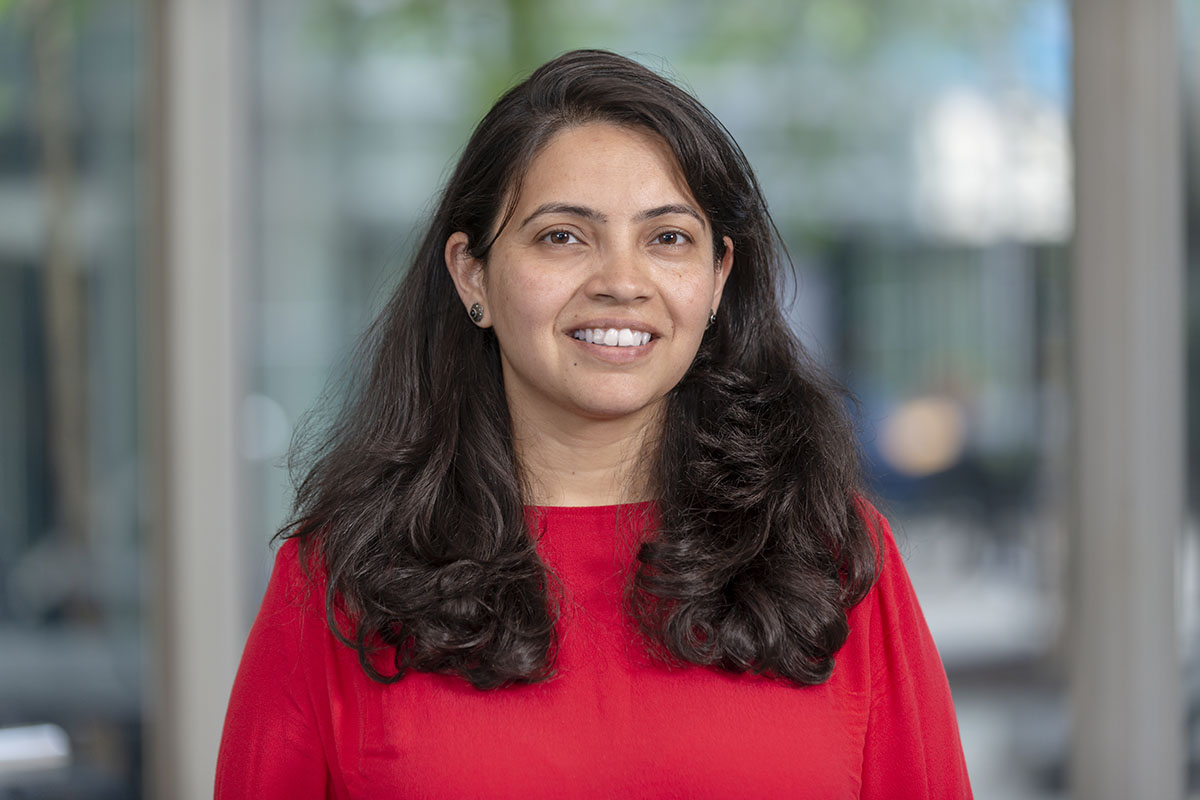
Rutu Pandya
Senior Programmer Analyst
Ms. Pandya has completed her Master’s in Computer Engineering and has been working on software development, testing, and deployment. At MSK she is involved in the development and transition of software tools from bench to bedside. Ms. Pandya also supports the development of CERR. Her latest work involves integrating auto-image segmentation algorithms using Deep Learning techniques, wrapped in singularity containers and integrated with CERR to be used in the clinic.
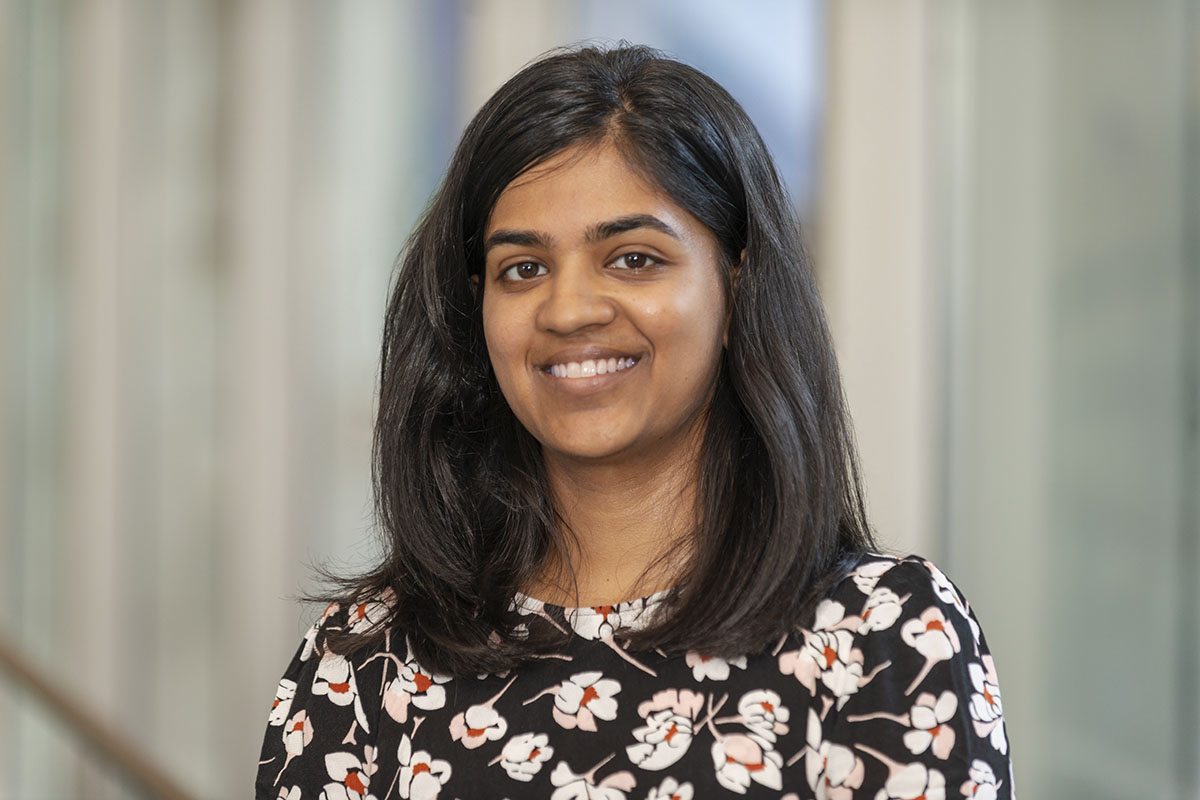
Aditi Iyer
Programmer Analyst
Ms. Iyer works on developing tools for image and dosimetry analysis available in CERR. Notable contributions include expanding CERR’s radiomics toolbox and developing the ROE interface for exploring the impact of prescription changes on local control probabilities. Ms. Iyer is also interested in the application of machine learning methods to medical image segmentation.
Research Study Technician
Ms. LoCastro performs computational modeling research with Dr. Shukla-Dave and develops new software for our lab’s quantitative image analysis package, MRI-QAMPER. She has extensive experience in designing and administering XNAT systems for data and research study management and has written integrated image segmentation and other custom post-processing pipelines for use in those servers.
She has prior experience establishing XNAT at Weill Cornell Medical College, New York:
XNAT is a widely used software platform for imaging informatics. It’s core functionalities include importing, archiving, processing and securely distributing imaging and the associated study data. XNAT’s access control system allows investigators to make their data openly accessible to users of their XNAT instance, accessible by request, or completely closed. Its support for anonymization and DICOM metadata review help ensure subject privacy and compliance with HIPAA regulations. XNAT provides tools to harmonize labeling of images. XNAT’s extensible data model allows investigators to share a variety of non-imaging data and derived image data with their imaging studies. XNAT allows users to develop and deploy plugins. The XNAT marketplace provides access to a variety of plugins that support data processing and non-dicom data types.
CQIBA will use XNAT as the central repository to share data between collaborators. An MSK-approved security mechanism will be used to secure the data. Singularity containers will be connected to XNAT processing pipelines to invoke analysis on data submission. XNAT database will house DICOM as well as the transformed/analyzed non-dicom data.