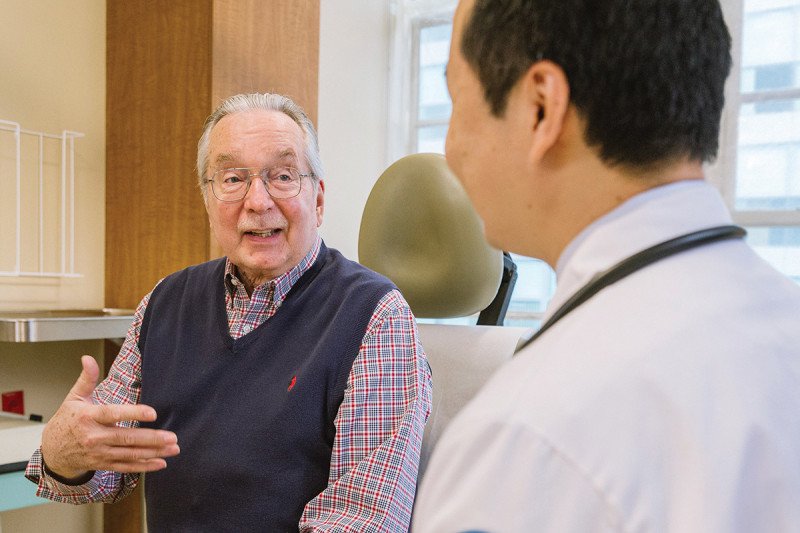
After genetic testing of his tumor, Albert Kuchler (left) enrolled in a clinical trial led by medical oncologist Bob Li. Photo: Kreg Holt
By Julie Grisham
It was July 17, 2017, when Albert Kuchler found an unusual lump in his neck. As a retired oral surgeon, he knew this was cause for concern. He called his friend and colleague Jatin Shah, a member of Memorial Sloan Kettering’s Head and Neck Service, to see if he could come in for an appointment. Dr. Shah was able to see him the very next day, and soon after, pathology tests revealed that Albert had salivary gland cancer.
Albert, now 66, was treated with radiation and surgery by a team of doctors, including Ian Ganly, Josh Yamada, Nancy Lee, and Loren Michel. Treatment went well for a few months, but then a scan showed that Albert’s cancer was spreading: first to his spine and hip, and then to his liver and lung. There was no obvious next step for treatment.
“Things were not going well for me at that point, to put it mildly,” Albert says. “The cancer was really aggressive.”
He understood how difficult this cancer was to control and how limited his options were for successful treatment. Salivary gland cancer is rare, and researchers are still learning what causes it to grow and spread.
Albert’s doctors analyzed his cancer with MSK-IMPACT™, a test that scans tumors for genetic changes known to play a role in cancer. They learned that Albert’s tumors were driven by HER2 amplification, causing an excessive amount of the HER2 protein to be present on tumor cells. This characteristic is usually associated with breast cancer and stomach cancer. Albert’s doctors promptly recommended that he see Bob Li, a medical oncologist and drug development scientist at MSK.
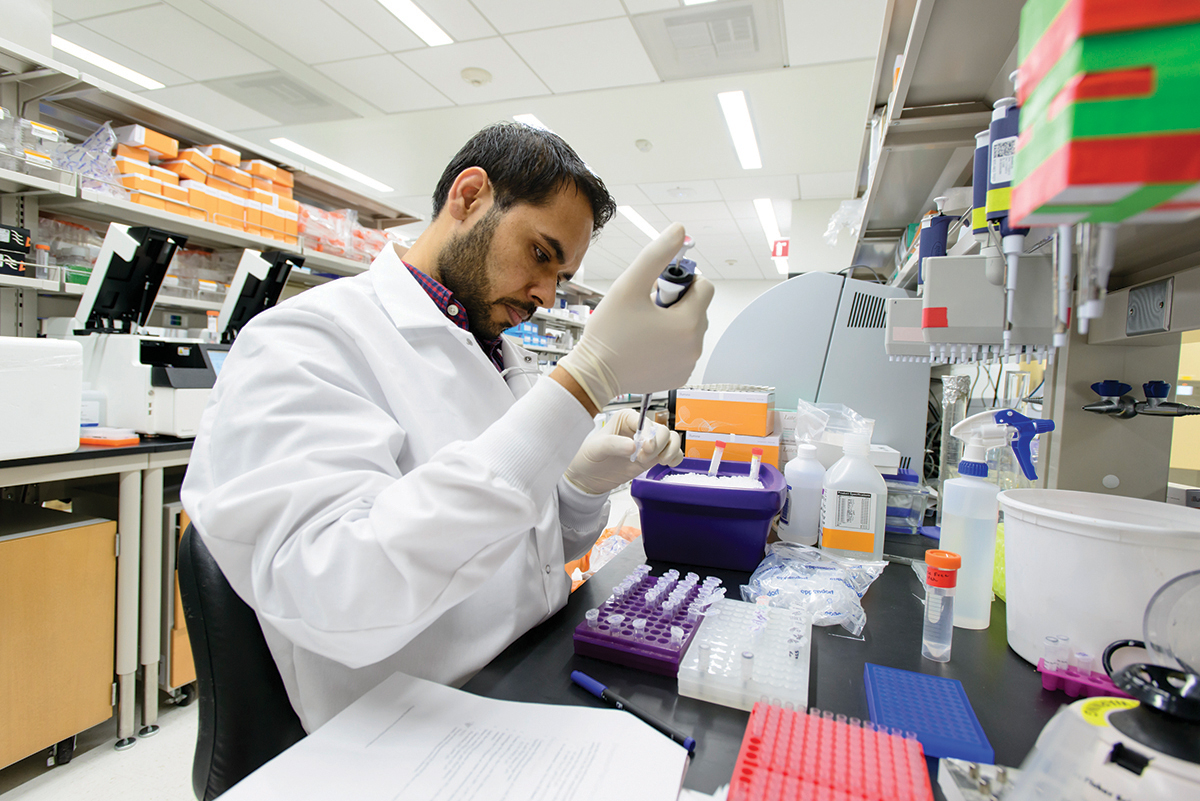
Operations engineer Ajay Sharma works in the MSK laboratory that developed MSK-IMPACT™. Photo: Rick DeWitt
“HER2 amplification is found in about 8 percent of salivary gland cancers,” says Dr. Li.
Albert now had a path forward. He enrolled in a clinical trial looking at a drug called ado-trastuzumab emtansine (Kadcyla®) in salivary gland tumors with high levels of HER2.
“The challenge of developing trials like this is finding more people with the right genetic mutation so that we’re able to offer them these potentially lifesaving treatments,” Dr. Li says. “Without MSK-IMPACT, we would not have found this new treatment for Albert.”
MSK-IMPACT uses cutting-edge, next-generation DNA sequencing to look for mutations and other critical changes in the genes of both rare and common cancers. The test has been used at MSK since 2014.
The Role of Machine Learning
MSK-IMPACT is possible because advances in computing and data science allow doctors to find mutations in thousands of cells. Once those mutations are identified, patients can be matched with the most-appropriate treatments.
Some 50,000 tumors have already been studied with MSK-IMPACT. The massive amount of data collected creates unique opportunities for analysis. One way to harness these data is by using artificial intelligence techniques, like machine learning. With machine learning, computers are trained to use large quantities of data to detect patterns and make predictions based on those patterns. Machine learning powers many things in our daily lives: Netflix recommendations, real-time driving directions, and more.
But while machine learning is playing an increasingly important role in medical decision-making, according to Sloan Kettering Institute computational biologist Quaid Morris, it is not a replacement for the judgment of doctors like Dr. Li and his colleagues.
“We employ artificial intelligence when there’s simply too much data for a human to look at,” says Dr. Morris, who recently was recruited to MSK to broaden the application of machine learning to new areas. “Machine learning helps make doctors better at their jobs.”
Learning about Tumors That Evade a Simple Diagnosis
Machine learning has the potential to further revolutionize patient care through new applications using MSK-IMPACT data. One of these applications is the development of diagnostic methods for tumors that are not easy to categorize.
In a study published in JAMA Oncology in November 2019 and led by cancer geneticist Michael Berger and computational oncologist Barry Taylor, MSK investigators reported on an algorithm that helps determine where in the body a tumor originated. This is possible, Dr. Berger says, because a cancer may have particular genetic changes depending on where it starts. Between 2 and 5 percent of all cancers are classified as cancer of unknown primary (CUP). This means that the place in the body where the cancer began cannot be determined.
Using machine learning, doctors can analyze and understand genetic patterns to figure out where a case of CUP started. According to Dr. Berger, even with treatments that target tumors based on their genetics, it’s still important to identify where a tumor started to be able to offer the most-appropriate drug.
“Machine learning has enabled us to develop extremely powerful new methods for diagnosing challenging cancers,” Dr. Taylor says. “We hope to validate more of these tools so they can be applied to patient care.”
“Contributions from donors have been very important in helping us establish these data sets,” Dr. Berger explains, “particularly philanthropy received through the Marie-Josée and Henry R. Kravis Center for Molecular Oncology and Cycle for Survival.” (Cycle for Survival is MSK’s indoor-cycling event, which raises money for rare cancer research.)
Getting a Better View inside the Body
Another way investigators are using machine learning is to improve PET scans, one of several imaging technologies used to diagnose cancer and determine if a treatment is working. These scans allow doctors to see activity in cells, including the rapid growth that distinguishes tumors from healthy tissue.
However, with current techniques it takes a long time to process the data that are generated with PET scans and to create images that can be interpreted by radiologists. Additionally, the images are sometimes blurry. MSK researchers have used a kind of machine learning called deep learning to look for a better approach.
“We wanted the computer to learn how to use data to construct an image,” says medical physics researcher Ida Häggström. “This represents a new way to process PET image data.”
Dr. Häggström and her colleagues, including medical physicist Ross Schmidtlein and data scientist Thomas Fuchs, named their technique DeepPET. According to a paper they published in Medical Image Analysis in May 2019, the method generates images more than 100 times faster than conventional techniques. The pictures are also much clearer than those made with current PET technologies.
The team is getting the system ready for clinical testing. “The gain we’ve seen in speed and image quality should lead to more-efficient image evaluation and more-reliable diagnoses and treatment decisions,” Dr. Häggström says. “Ultimately, it can result in improved care for our patients.”
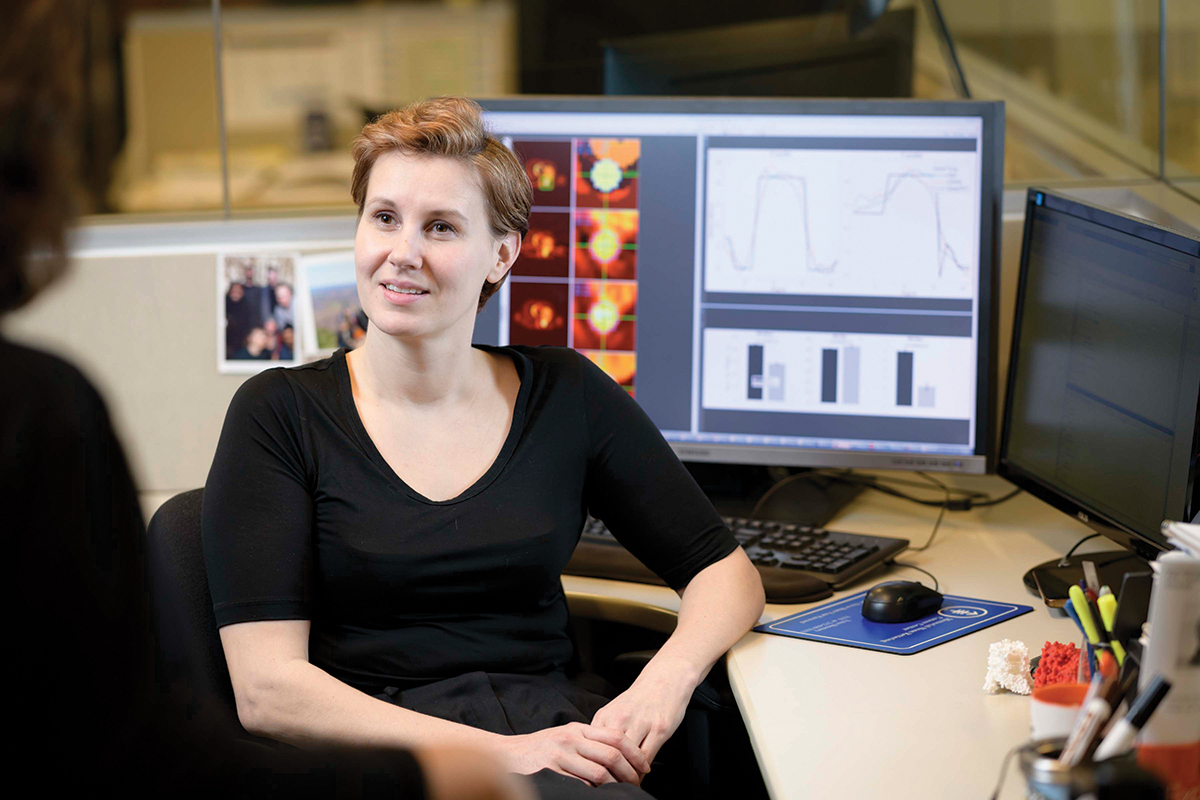
Medical physics researcher Ida Häggström is using a technology called deep learning to improve PET scans. Photo: Rick DeWitt
New Research Methods, New Challenges
Machine learning is useful for more than making sense of data sets from patients. It can also parse data sets from individual cells being studied in the laboratory.
How do immune cells and tumor cells interact? Which molecular changes enable tumors to spread beyond the organ where they originally formed? These are just some of the questions being addressed by Dana Pe’er, Chair of the Computational and Systems Biology Program in SKI. Dr. Pe’er is a leader in developing machine learning algorithms to address fundamental questions in biomedical science, including how to analyze the huge amount of data that comes from analyzing the molecular information in every cell in a tissue sample as part of lab research.
Dr. Pe’er collaborates with investigators throughout SKI and the rest of MSK to help researchers make sense of complex molecular changes and figure out which ones are significant.
In one recent study, published in Nature Medicine, she and SKI Director Joan Massagué led a team that found uncanny parallels between lung cancer cells that are able to metastasize (spread) to other parts of the body and the stem cells involved in lung development and wound repair. The investigators analyzed genetic information from 40,000 individual cells from 17 tissue samples taken from patients. They then used advanced computational methods developed in Dr. Pe’er’s lab to interpret this head-spinning amount of data. The findings lend support to the idea that a metastasis can be viewed as a dysfunctional organ that grows in the wrong place.
Sohrab Shah, MSK’s Chief of Computational Oncology, is also using machine learning to address the flood of information that results when cells are studied one at a time.
In a study published in Cell in November 2019, he and his collaborators sequenced the genomes of nearly 52,000 individual cells and analyzed how these genomes changed over time. This allowed them to pinpoint the mutations that gave rise to a particular tumor and determine how certain groups of cancer cells evolved.
Understanding how tumors change over time is essential to finding ways to detect them early and stop their growth sooner. The study of tumor evolution is also important for learning how cancer cells develop resistance to treatment. In the future, these applications have the potential to further revolutionize cancer care.
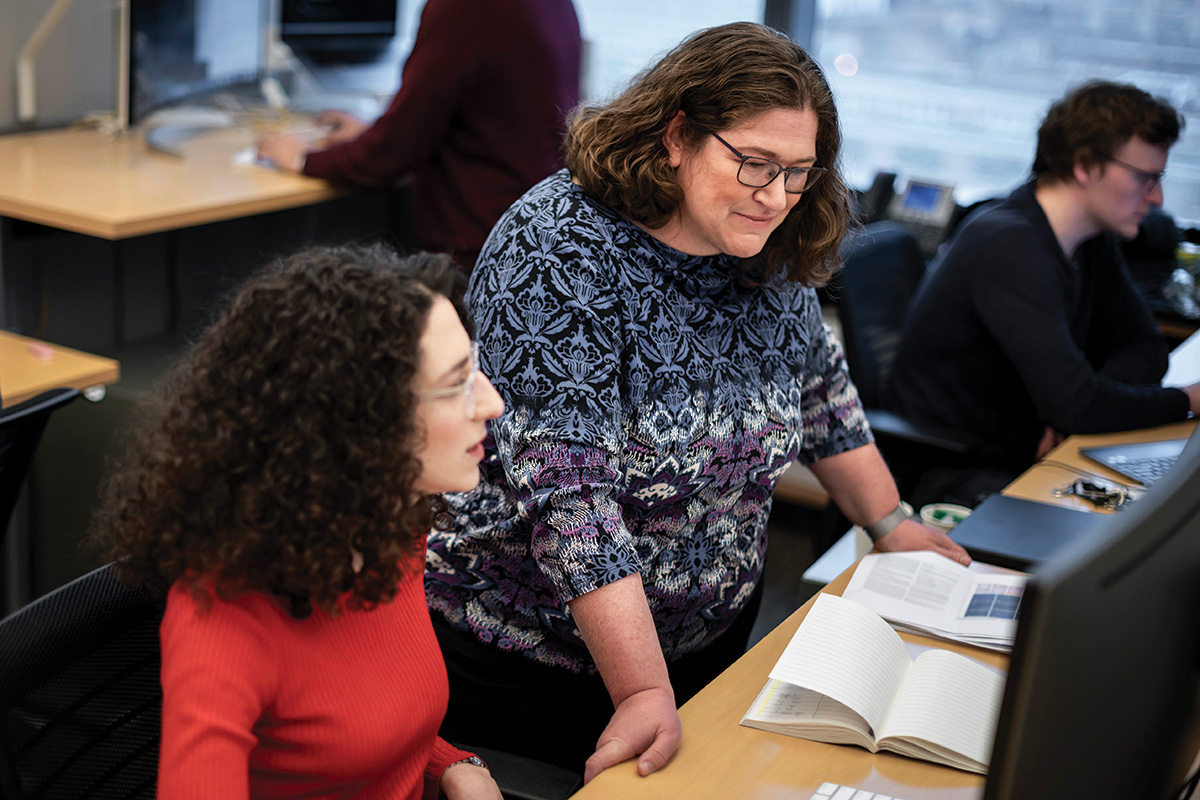
Computational and Systems Biology Program Chair Dana Pe’er (right), here with research scholar Esther Wershof, is a leader in developing computer algorithms to make sense of data from single-cell analysis. Photo: Karsten Moran
Using Innovative Technology Today
As Albert’s story demonstrates, however, the power of computational biology and data science is already here.
After just two infusions of ado-trastuzumab emtansine, all of his tumors completely vanished, and his bones were healing. “My scans were remarkable, to say the least,” Albert says. “My response to the drug was as good as you could possibly get.” More than a year later, he shows no evidence of disease.
Albert says side effects from the treatment have been minimal. He will continue to receive infusions of the drug every three to four weeks for as long as it works.
Once an avid golfer, Albert is not quite ready to take up his clubs because his spine is still healing, but he has returned to his golf-related volunteer work — he acts as a referee at United States Golf Association amateur tournaments across the country and at New Jersey State Golf Association professional and amateur tournaments. “It’s been great to get off the hamster wheel of treatment and start to participate in my hobbies again,” he says. “I’m very grateful for the opportunity to participate in this trial.”