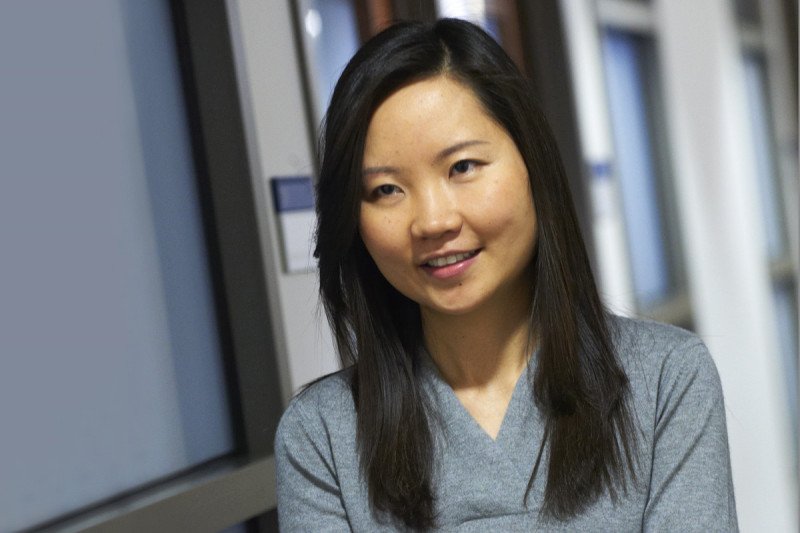
Current Research Interests
Dr. Shen’s research interest lies in developing statistical and computational genomics approaches and their applications to translational cancer research. She developed iCluster, a statistical data integration method for defining molecular subtypes of cancer and associated biomarkers across multiple “omic” data types simultaneously characterizing genomic, epigenomic, transcriptomic, and proteomic aberrations in a tumor. This method has been widely used in the NCI-NHGRI Cancer Genome Atlas (TCGA) project, with many of the primary analyses involving individual cancer sites that were published in a series of articles in Nature employing the method. Together with Dr. Venkatraman Seshan, she developed FACETS, an algorithm that can be used to explore allele-specific copy number aberrations and clonal heterogeneity within a tumor using whole-genome, whole-exome and targeted capture sequencing data. Her recent research interest also includes a novel investigation of somatic variant richness using statistical methodologies developed in ecology and computational linguistics, joint work with Drs. Saptarshi Chakraborty and Colin Begg. This project uses sophisticated statistical tools to extract information from rare variants in existing databases with a view to identifying the site of origin for cancers of unknown primaries and cancers detected from circulating cell-free DNA in the blood. With Drs. Margaret Callahan and Katherine Panageas, she is co-leading a biomarker development effort in linking peripheral blood immune cell phenotype from flow cytometry analysis with clinical outcomes in patients treated with immune checkpoint inhibitors.
My Downloads
Disclosures
Doctors and faculty members often work with pharmaceutical, device, biotechnology, and life sciences companies, and other organizations outside of MSK, to find safe and effective cancer treatments, to improve patient care, and to educate the health care community.
MSK requires doctors and faculty members to report (“disclose”) the relationships and financial interests they have with external entities. As a commitment to transparency with our community, we make that information available to the public.
Ronglai Shen discloses the following relationships and financial interests:
No disclosures meeting criteria for time period
The information published here is for a specific annual disclosure period. There may be differences between information on this and other public sites as a result of different reporting periods and/or the various ways relationships and financial interests are categorized by organizations that publish such data.
This page and data include information for a specific MSK annual disclosure period (January 1, 2022 through disclosure submission in spring 2023). This data reflects interests that may or may not still exist. This data is updated annually.
Learn more about MSK’s COI policies here. For questions regarding MSK’s COI-related policies and procedures, email MSK’s Compliance Office at [email protected].
View all disclosures